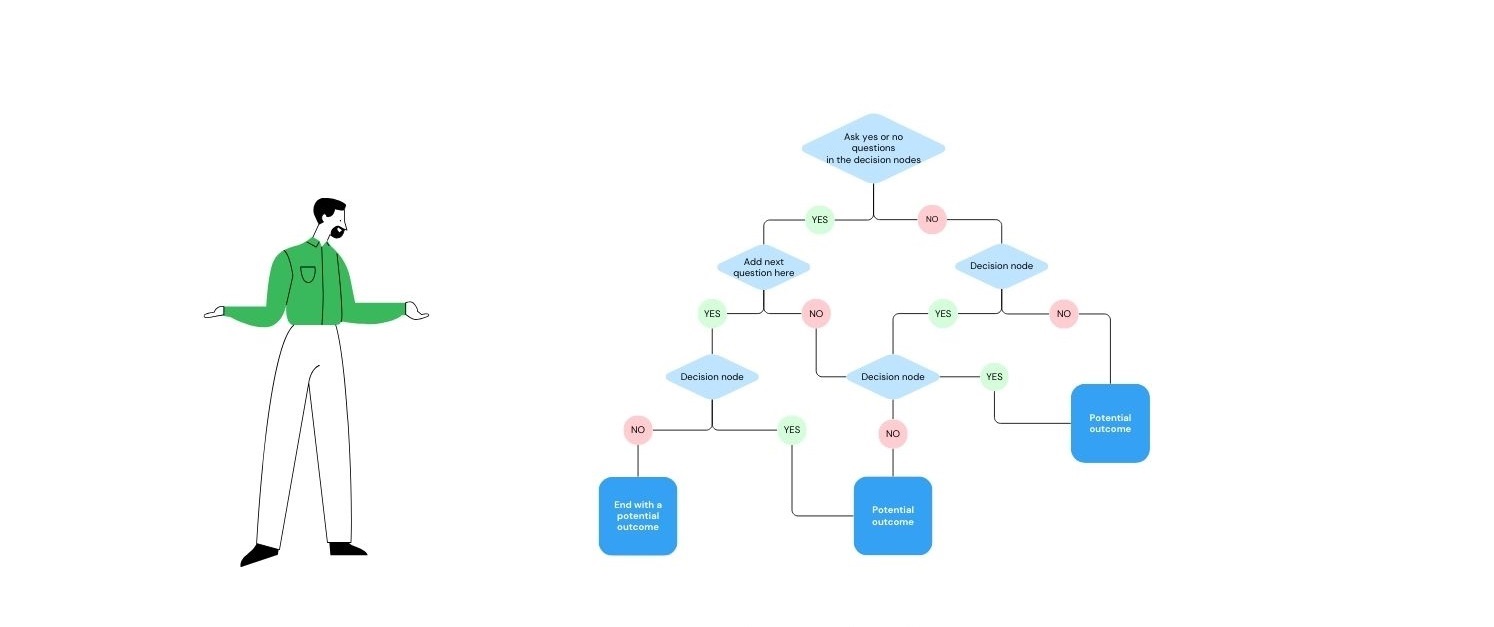
KKR-HR Joint Venture: Case Study on deploying AI in Commercial Real Estate for signal spotting
The commercial real estate (CRE) market is brimming with potential for AI-driven insights. However, a significant obstacle exists: fragmented data and a lack of transparency. Large language models (LLMs) excel in many fields, but in CRE, their effectiveness is hampered by incomplete information. We certainly recognize that LLMs alone are insufficient for navigating the complexities of CRE.
This is why we're spearheading an initiative: the creation of a gold standard dataset designed specifically to train and empower AI models tailored to the CRE landscape. We believe this dataset will be a game-changer for academics, data science professionals, and AI enthusiasts like yourselves.
The Data Chasm: AI Needs Grounding in CRE
The current state of CRE data creates a significant hurdle for AI. Ownership details, property specifics, and leasing information often reside in isolated silos. This hinders the ability of LLMs to grasp the intricate nuances of the market, potentially leading to inaccurate predictions and missed opportunities.
We are actively tackling this challenge. By meticulously gathering and organizing CRE data, we're building a comprehensive and standardized dataset unlike any other. This allows us to leverage the power of human expertise alongside advanced data analysis, achieving a level of market insight that attempts to overcome the challenges faced with current AI models.
A Case Study: Leveraging AI for the KKR-HR REIT Medical Office JV
Let's take a recent example: the announcement of a $1 billion joint venture (JV) between KKR and Healthcare Realty Trust (HR REIT) targeting medical office buildings in major markets [Commercial Observer, KKR Partners With Healthcare Realty Trust on $1B Venture].
While the official property list remains undisclosed, we employed our proprietary database as experimentation with OpenAI's and Google's Gimini LLM models to identify the most likely candidates for the JV. This prediction wasn't solely based on intuition; it was fueled by the power of meticulously curated CRE data, which is provided to the LLM as context so that results are grounded in proper facts. Simply put, we attempted an academic experiment into the accuracy of predictability algorithms used by LLMs when they’re supplied with better context data. In our case, it was the full portfolio of HR REIT so the LLM no longer had to figure out what was owned by the REIT because we provided that context. We further provided “Market” and “Submarket” data, so the LLM could align with the narrative of the press release to make its predictions more accurately. While we thought of extrapolating this exercise to other properties without our “medical office” database to recommend likely contenders for investment, we stopped at Step 1, to learn and reflect before moving to Step 2.
Based on our academic experiment, the likely candidates for the KKR-HR REIT Medical Office JV from within the existing REIT portfolio are:
Property Name | Address | City | State | Submarket* | Market* |
2061 Peachtree | 2061 Peachtree Road | Atlanta | GA | Atlanta: Buckhead | Atlanta |
Illinois Masonic POB | 3000 North Halsted Street | Chicago | IL | Chicago: Near North Suburbs | Chicago |
ProMed Westchester Medical Campus | 244 Westchester Ave | White Plains | NY | New York: Westchester County NY | New York |
Civic Center Place | 39141 Civic Center Dr. | Fremont | CA | San Francisco: East Bay_Fremont_Newark_Union City | San Francisco |
MedStar POB North Tower | 106 Irving Street NW | Washington | DC | Washington DC: Northern DC_I-295 | Washington DC |
Fair Oaks MOB II | 3700 Joseph Siewick Drive | Fairfax | VA | Washington DC: Northern VA_Reston_Herndon | Washington DC |
Fair Oaks MOB I | 3650 Joseph Siewick Drive | Fairfax | VA | Washington DC: Northern VA_Reston_Herndon | Washington DC |
This experimental case study exemplifies the immense potential of AI in CRE, when empowered by high-quality contextual data. However, we recognize that AI for CRE is still in its early stages and that it may be many months before the assets in this JV are disclosed publicly.
This experiment was our initiative to engage you, the data science and AI community to collaborate with us. RealAssetData is open to your ideas on collaborating for the greater good. We believe that by sharing our gold standard dataset and fostering a collaborative environment, we can unlock the true potential of AI in CRE.
Are you an academic, data scientist, or AI enthusiast eager to push the boundaries of CRE analysis? We invite you to reach out and explore how our gold standard dataset can fuel your next groundbreaking project. Together, we aspire to bridge the data gap and usher in a new era of AI-driven foresight in the CRE market.
-------------------------------------------------------------------
*Market and Submarket boundaries are proprietary geospatial datasets we use to classify all properties within our database.
-------------------------------------------------------------------
-------------------------------------------------------------------
Disclaimer: RealAssetData and its information providers are not liable for any delays, content errors, or actions based on this report. The data is for informational purposes only and does not constitute investment advice or an offer to buy/sell securities. Redistribution of content is prohibited without written consent. See our Terms of Use and Privacy Policy at www.realassetdata.com.